Table of Contents
- The AI Industry1-1. AI and its origins1-2. Key to AI development: Data processing1-3. Concerns that arise from AI development
- Blockchain, The Answer to AI Limitations
- Notable AI Sector Projects3-1. Worldcoin offers new solutions using inherent nature of blockchain technology3-2. NEAR Protocol, the L1 network for self-sovereignty3-3. Bittensor builds P2P AI market to address high entry barrier and long tail issue
- What Challenges Are AI and Blockchain Facing?4-1. Scalability, scalability, scalability
- Final Thoughts
Introduction
In March 2024, the price of Bitcoin surged, surpassing its peak from 2022. This bullish trend is attributable to factors such as the approval of Bitcoin spot ETFs, institutional capital inflow, the upcoming Bitcoin halving in April, and anticipation of U.S. interest rate cuts. Consequently, optimism for price increases in the entire crypto asset market has spread, with many investors diving into crypto investments. Historically, Bitcoin halvings come around every four years and showed a strong uptrend of Bitcoin, accompanied by sectors leading the market alongside it. During the halving in 2020, there was a significant uptrend in decentralized finance (DeFi), resulting in what was termed the “DeFi Summer.” As a result, we’re seeing crypto investors seeking sectors that will lead the market in line with the 2024 Bitcoin halving. The most promising sector currently is AI. As of March 13, projects like Worldcoin, launched by Sam Altman, considered the father of AI, surged by 304% in just a month. NEAR Protocol, founded by Illia Polosukhin, laying the foundation for generative AI, surged by 123% in just a month. Moreover, Bittensor, with its goal of establishing a P2P AI market through decentralized AI training platforms, witnessed an approximate 810% surge over a year. So why is the AI sector getting such attention, and what potential does the market see in AI? Let’s delve into why the AI sector is gaining attention in the crypto asset market and speculate what the future holds for it.
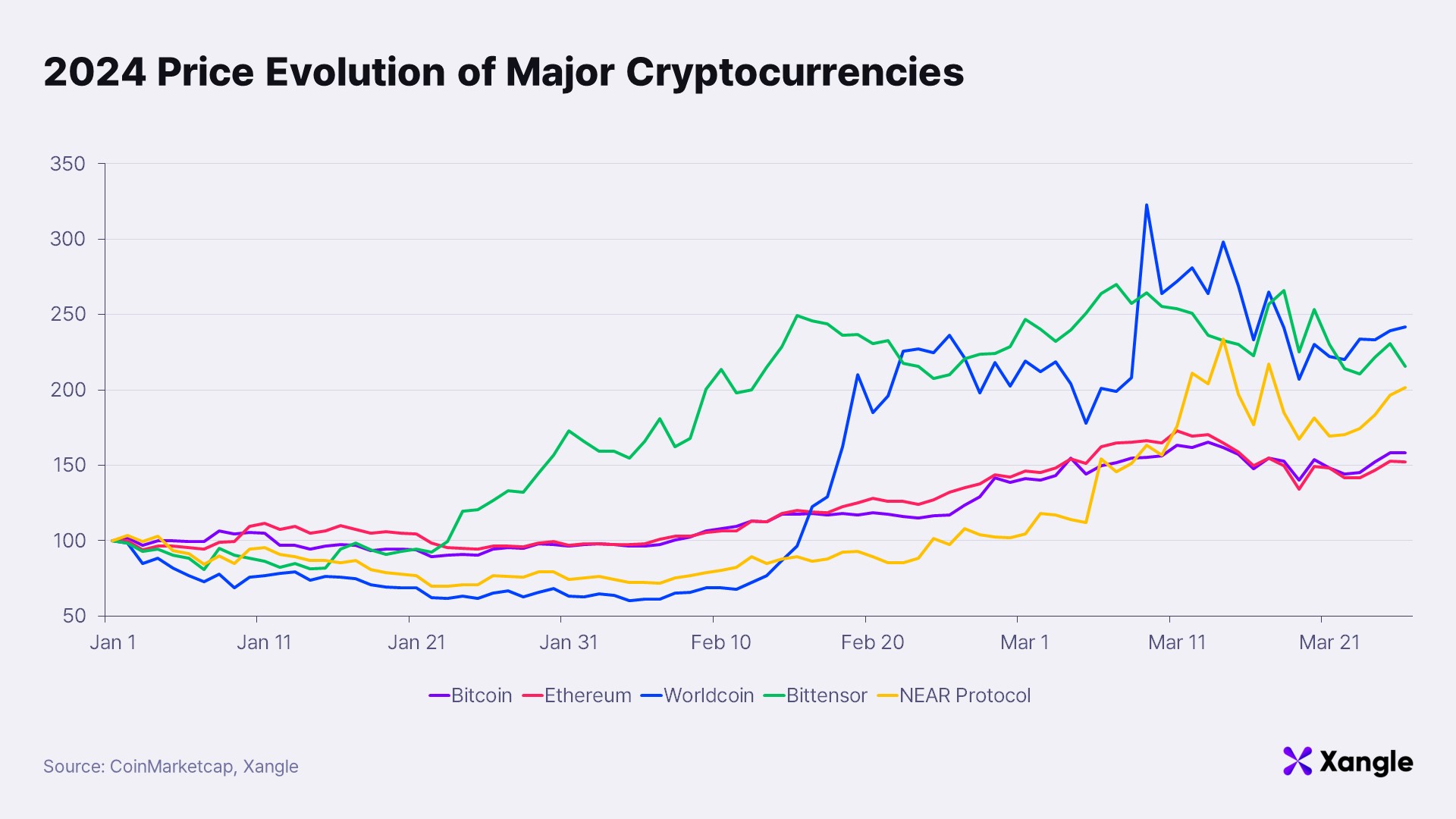
1. The AI Industry
1-1. AI and its origins
Artificial Intelligence (AI) is a field of computer science that aims to mimic human intellectual abilities such as learning, reasoning, problem-solving, cognition, and language understanding using computer programs.
The history of AI dates back to the 1950s. In the year 1950, Alan Turing, a British computer scientist, proposed the concept of “computing machinery and intelligence” and introduced the Turing Test (a method that determines whether a machine can demonstrate intelligent behavior that is equal to or discernible from that of humans), questioning whether machines can think like humans. In 1956, the term “AI” was first coined at the Dartmouth Conference, attended by John McCarthy, Marvin Minsky, Allen Newell, Herbert Simon, among others. This conference is considered the formal beginning of AI research and laid the groundwork for machine learning, data mining, and other fields.
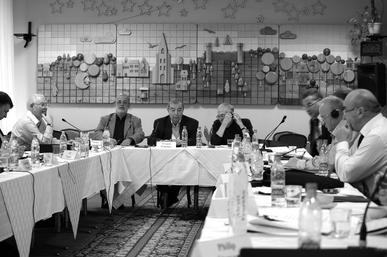
Source: Wikipedia
1-2. Key to AI development: Data processing
Initially proposed in the 1950s, AI began to be utilized in rule-based systems in business and medicine in the 1980s. As AI started to take over some of the human roles, concerns arose about AI potentially fully replacing humans—a concern that still persists today.
However, as of 2024, AI has not reached the level of technological advancement that was anticipated, and attention is now turned toward General Artificial Intelligence (AGI). In other words, a gap exists the initially expected and the actual pace of AI development. So why did it take so long for AI reach the current level of development and what are some of the factors that influenced it?
To understand this, we need to touch upon the advancement of data processing devices used in AI. In the 1980s and 1990s, the speed and processing power of the central processing unit (CPU) of computers improved rapidly. Multi-core processors (CPUs with more than two independent cores on a single chip) emerged during this period, enabling computers to perform multiple tasks simultaneously, leading to a significant improvement in computer performance and the beginning of popularizing computers.
Subsequently, the graphics processing unit (GPU) was released in the 1990s and became popular in the 2000s, further advancing the computer and AI markets. GPUs process data in parallel, making them unsuitable for processing highly complex computations, but they are useful for processing a large amount of data simultaneously. As GPUs became widespread, they significantly influenced computer graphics screens, which require processing of thousands of pixels of data, and AI development, which requires learning from billions of data. AI has developed alongside the advancement of data processing capabilities, and recently, high-bandwidth memory (HBM) is gaining attention again for enhancing GPU’s data processing capabilities.
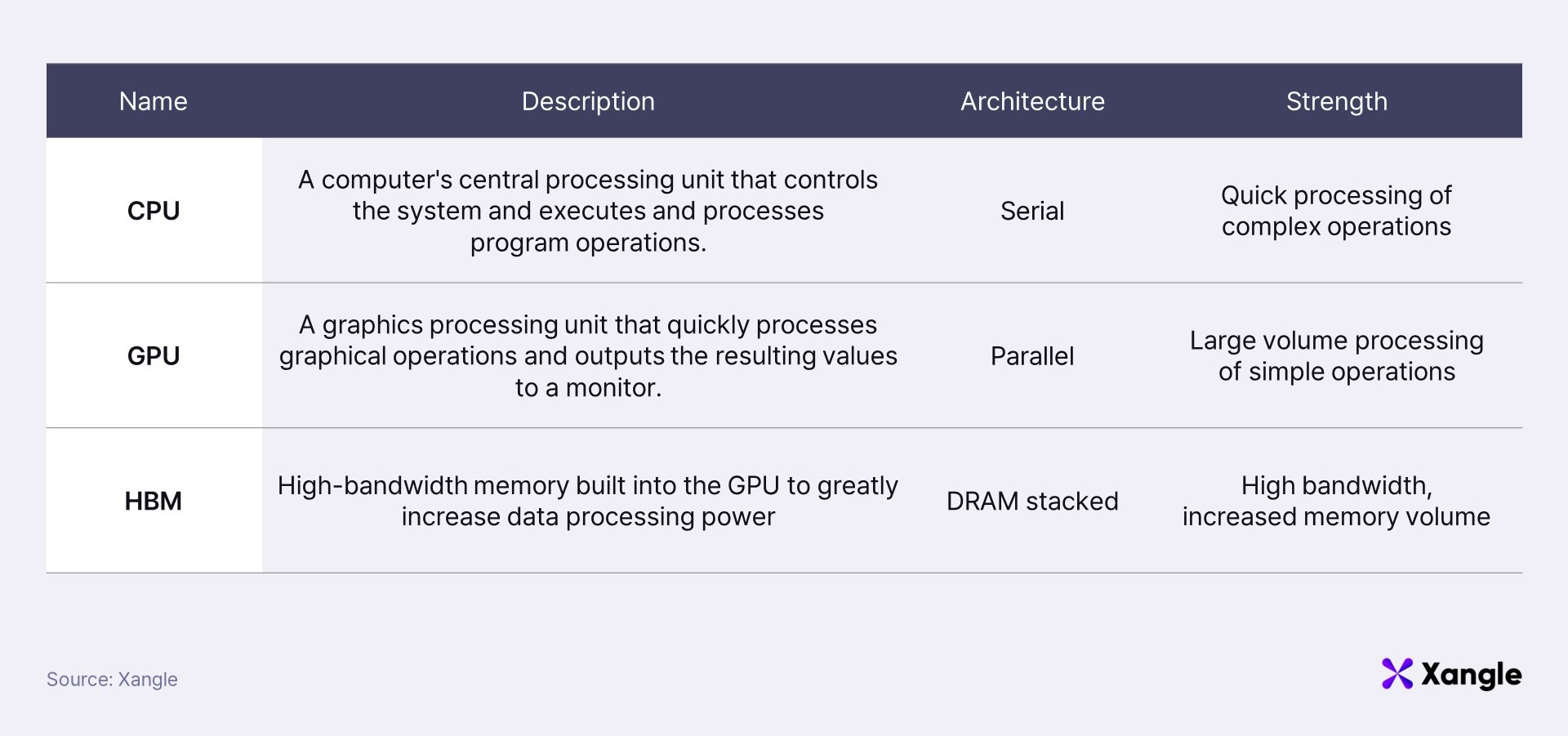
1-3. Concerns that arise from AI development
The AI market has rapidly developed with the improvement in data processing capabilities. Particularly, the generative AI market began to grow with the advent of GPUs enabling deep learning and natural language processing. ChatGPT is an exemplary case as an interactive AI chatbot developed by OpenAI in 2022. It demonstrates remarkable abilities across diverse domains such as question answering, translation, and research compared to humans, outpacing humans and heralding the age of generative AI.
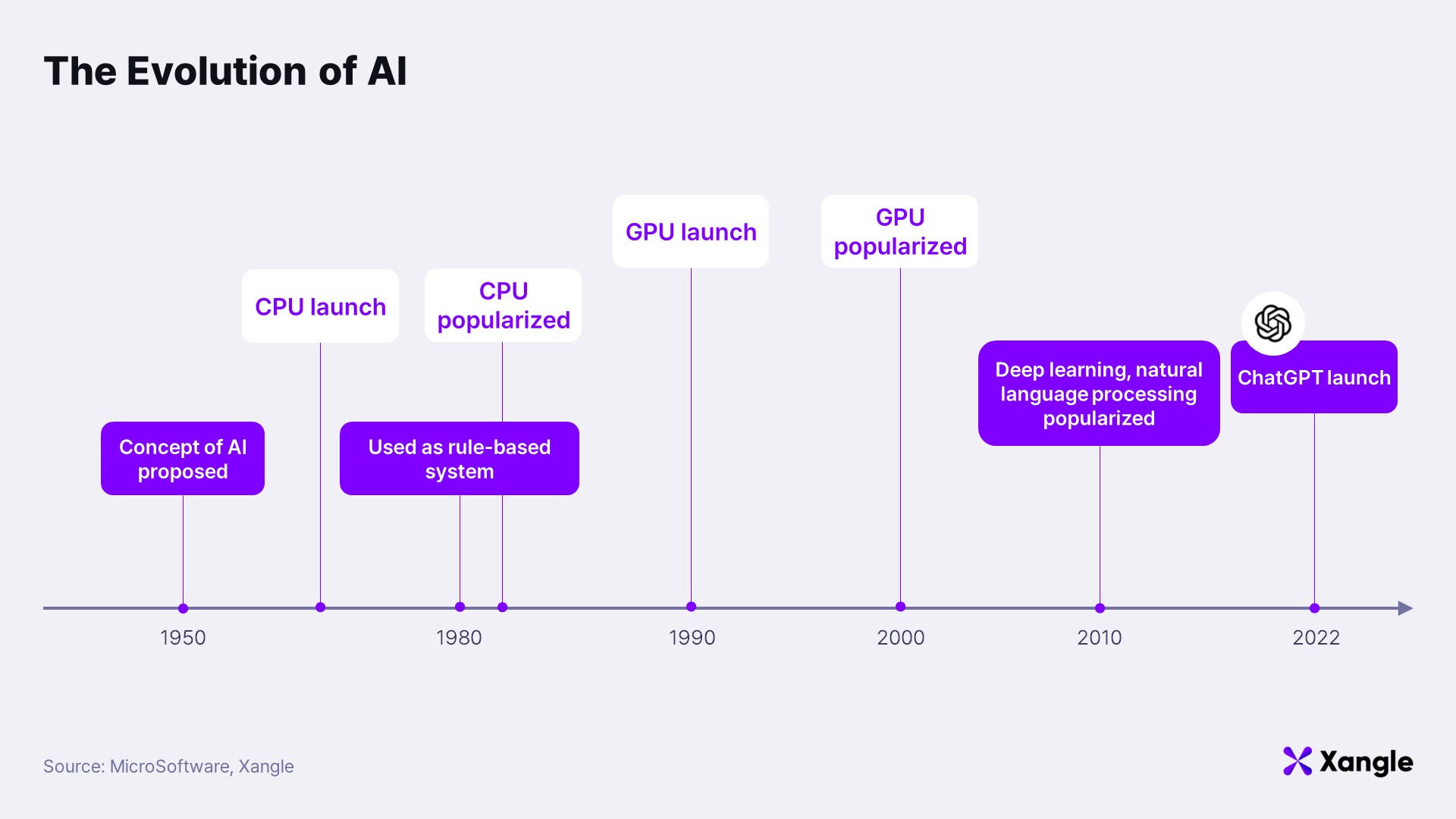
However, concerns about generative AI grew as it began surpassing humans in efficiency when performing tasks. Generative AI relies on learning from vast amounts of data and is currently managed by centralized entities—raising issues such as data transparency, long tail problems, and sovereignty. Moreover, instances of criminal misuse have been reported, including the creation and distribution of fake news or mass production of malicious code using ChatGPT’s capabilities. Consequently, longstanding criticisms of AI have heightened, fueling ongoing discussions about the potential problems AI may bring continue. OpenAI CEO Sam Altman even mentioned the potential risks of AI, emphasizing the importance of understanding the impact of AI technology on society and appropriate regulation. Flaws in AI industry often discussed are as follows:
- Long Tail: It is the phenomenon where the AI’s performance diminishes for certain data due to biased data that the AI learns from. Since the AI relies on large amounts of data, biased data can lead to the AI’s biased judgment. This becomes a more significant issue, particularly when small amounts of unstructured training data are provided, requiring prolonged periods and trial and error to secure performance.
- Data Opacity: Generative AI derives results based on learned data. Consequently, there is a possibility that results may include incorrect information or data that infringes on copyrights. However, since current generative AI uses a centralized method of data management by companies, users cannot know which data the results are based on or what data the AI service has learned. Furthermore, as generative AI produces proposes results based on data learned through complex algorithms, it is technically impossible to perfectly capture what data influenced the result. We call this the “black box phenomenon.”
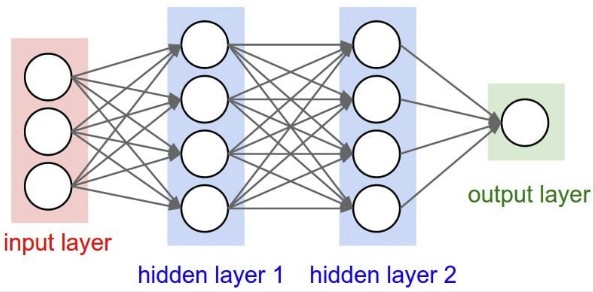
Source: BMC
- Data Sovereignty: As of June 2023, the top three ranking generative AI in terms of global user count are ChatGPT, Character.AI, and Bard, which are all U.S. companies. Generative AI has primarily developed around American global fintech companies, and most users are concentrated there. Consequently, data sovereignty lies with a few American companies, raising the possibility of control by the U.S. government or corporations.
- High Entry Barriers: To create generative AI models, high computational power is required, and the process involves learning billions of data at least. Therefore, the cost is very high, making it difficult for companies to enter the AI industry.
- Criminal Misuse: Generative AI is adept at handling simple and repetitive tasks and can produce content at a level similar to humans. Recently, there have frequently been cases where malicious content or malicious code have been produced in mass using generative AI for criminal use.
2. Blockchain, The Answer to AI’s Limitations
As concerns over AI grow, blockchain technology has emerged as a key solution to address them. Blockchain, a technology that stores information across a distributed network, maintains data integrity and security without central management. Each data block is linked in a chain, making alterations impossible and ensuring transparency since all participants can verify the transaction records. Utilizing blockchain can enhance the data processing and management of AI, making it safer and more transparent, thereby resolving various issues associated with AI. And numerous efforts are currently underway to seamlessly integrate blockchain and AI. Some of the most anticipated advantages of integrating blockchain and AI are as follows:
Addressing accessibility, data sovereignty, and long tail
Integrating blockchain with AI can create a permissionless market where anyone can contribute, decentralizing the technological power concentrated in a handful of large corporations. The blockchain network is freely accessible, allowing anyone to contribute computing power or datasets and receive rewards, and anyone can use the provided datasets to build AI models. The blockchain network can also secure a variety of data for learning and solve long tail problems through token incentives that encourage market participation.
Better reliability and transparency
Blockchain stores transaction records in an unalterable form, providing transparency. Therefore, when blockchain and AI are combined, the data used for AI learning becomes transparently available to everyone, thus addressing the issue of data opacity. Ensuring data transparency can also prevent the misuse of AI in criminal activities.
Additionally, AI learns from users’ behaviors and preferences to offer personalized experiences. Currently, centralized corporations manage user data, raising concerns about potential privacy breaches. However, blockchain stores data across decentralized nodes and does not require registration of personal information like email addresses, eliminating the risk of privacy threats from centralized entities.
3. Notable AI Sector Projects
3-1. Worldcoin offers new solutions using inherent nature of blockchain technology
Worldcoin ($WLD) is a project launched in July 23 by Sam Altman, CEO of OpenAI, the developer behind ChatGPT. Worldcoin aims to distinguish between humans and AI by utilizing iris recognition in preparation for the AI era and seeks to establish a global economic community. Users of Worldcoin can register their iris information through the iris recognition device called the Orb and receive World ID and Worldcoin ($WLD) as digital identity and rewards.
- To read more about Worldcoin, refer to “WorldCoin & Friend.tech: Steps in the DApp Evolution”.
Worldcoin gained attention when it surged by nearly 304% in just one month following OpenAI’s release of SORA in February. With AI generating results that are increasingly difficult to distinguish from human work, Worldcoin’s initiative use iris data to recognize between AI and humans garnered attention. The snapshot of a video below features a woman that looks similar to a real person. The scene was generated based on extensive data learned by SORA, and it’s impossible to discern whose data was used or if anyone’s photo was exploited. Moreover, it’s impossible to determine if the video was captured by a human or generated by AI.
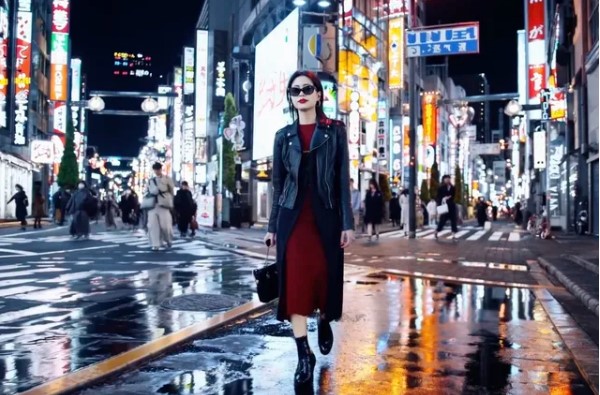
Source: OpenAI
In the era of AGI where the boundary between humans and AI becomes blurred, Worldcoin appears to be emerging as a digital identification. As AI advances, issues such as copyright infringement and human rights violations are expected to become more prevalent, especially with the rising risk of criminal activities employing technologies like deepfakes.
In fact, controversy arose in May 2023 when a then-Twitter account posted a photo of an explosion at the Pentagon, purportedly created by AI. This caused temporary turmoil in the U.S. stock market, which proves an urgent need to distinguish between AI and humans. Imagine the reverse scenario: You witnessed a fire at the Pentagon and took a photo to share on social media in order to alert others. However, no one believes it’s genuine and instead accuses it of being fake news generated by AI. In that case, how could you prove the authenticity of the photo?
As the development of AI blurs the boundary between humans and AI, proving human identity becomes essential. This raises Worldcoin’s significance in its endeavor to find solutions for these new era problems by leveraging blockchain’s unique features.
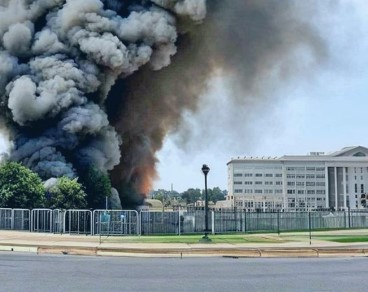
Photo of explosion at Pentagon, generated by AI (Source: X)
3-2. NEAR Protocol, the L1 network for self-sovereignty
NEAR Protocol ($NEAR) is a layer 1 project designed with a vision to achieve blockchain mass adoption by prioritizing usability for end-users and developers. Introduced by Illia Polosukhin, a co-author of the groundbreaking “Attention Is All You Need” paper and former project lead at Google’s deep learning group, in 2017, presenting the transformer neural network architecture*. NEAR consistently demonstrated AI-friendliness from its early days and Polosukhin himself emphasized the importance of AI and its applications in 2023. Recently, his participation as a speaker at GTC 2024, the world’s largest AI conference hosted by NVIDIA, also garnered attention.
- For more details on NEAR, refer to “The NEAR Ecosystem Set to Take Off in 2023”.
*The Transformer neural network architecture is an AI neural network that utilizes an attention mechanism that allows the model to emphasize certain parts of an input sequence so that those parts have a greater impact on the output, allowing it to better understand and interpret the overall context. The Transformer neural network architecture can process all the words in an input sequence at once, making it extremely fast and capable of capturing relationships between words over long distances. It is the basis of many natural language processing models such as GPT and BERT.
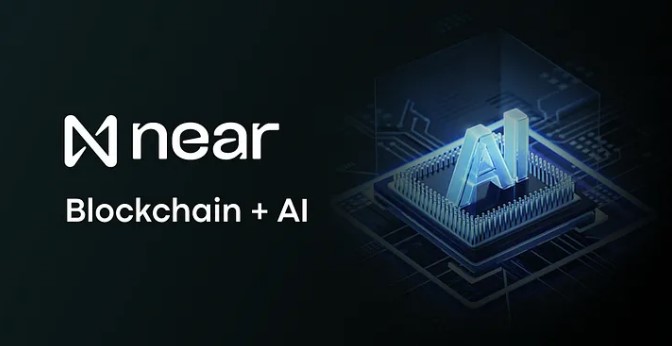
Source: NEAR Protocol
NEAR Protocol presents self-sovereignty as its vision, meaning individuals have control over their own sovereignty. Currently, AI optimizes algorithms based on user data to offer personalized content and products all thanks to data learning. However, centralized control means individuals lack sovereignty over their own data. In this setup, there’s a high probability that data could be commercialized, benefiting giant tech corporations. Moreover, if governments gain access to this data, they could use it to maintain or expand their power. Therefore, it’s crucial for individuals to have sovereignty over their data to prevent its misuse.
Securing self-sovereignty in data, therefore, is essential as AI usage increases. Every user should have control over their assets, data, and right to governance, as well as data sovereignty to be aware of how their data is being used. And Web3 systems can maintain, expand, and manage such systems, preventing AI misuse while enabling its positive impact.
NEAR aims to combine blockchain and AI to become a fully sovereign operating system with a personal AI assistant that optimizes for your needs while maintaining privacy about your data or assets. While we often see examples of AI being used in specific parts of protocols, it’s rare to see a vision for active AI use on the mainnet itself. This is where NEAR comes in, as it owns the data sovereignty and aims to provide a well-rounded and efficient experience through personalized AI. It will be interesting to see how NEAR will incorporate AI into its mainnet and what kind of UX that will bring forth.
3-3. Bittensor builds P2P AI market to address high entry barrier and long tail issue
Bittensor ($TAO) is a blockchain-based machine learning and AI training platform that aims to create a P2P traded AI market via blockchain, and it aims to achieve this through decentralized subnets. These subnets form separate networks where various AI development activities such as AI model creation, data collection, and computation can occur. Subnets are built with competition-based incentive systems, allowing anyone to provide the necessary datasets for AI training and receive incentives. The components and roles of the Bittensor subnets are as follows:
- Validators: Validators distribute rewards across subnets and validate miners’ work within each subnet. The validation of miners’ work varies from subnet to subnet and is based on benchmarks set by the subnet creator to ensure quality and relevance. Validators can sell AI models via APIs, similar to the business models of OpenAI, Anthropic, and Microsoft, without owning the entire network infrastructure.
- Miners: Miners earn incentives by performing tasks assigned to them on the subnet, such as providing computational power, developing new models, or training AI.
- Incentives: Each subnet has its own reward algorithm that focuses on speed (latency), accuracy, or a combination of metrics. Miners continuously optimize the software and hardware to earn incentives.
- Users: End users can use the product without any knowledge of AI, similar to using OpenAI or Google’s AI model.
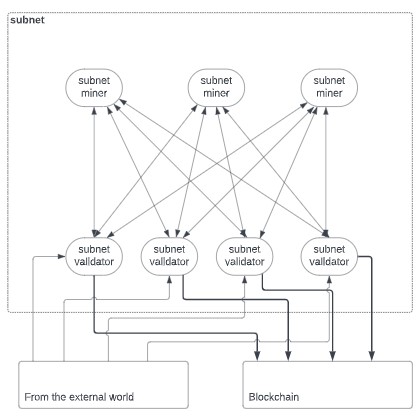
Source: Bittensor
By doing so, Bittensor aims to solve the long tail problem of data and build a peer-to-peer AI marketplace that anyone can participate in. As mentioned above, the generative AI market is currently dominated by U.S. fintech companies. Building generative AI requires a lot of capital, as it needs to an extensive amount of data to learn from. This is why the market has a high entry barrier for startups. Bittensor, however, provides an AI marketplace where anyone can contribute and participate. The token incentive allows anyone to contribute datasets, computing power, and rewards to the AI marketplace, which prevents data from being skewed or quality data from being marginalized. The tendency to being often skewed in favor of a few companies is an issue that AI must resolve. Thus, Bittensor’s idea is to build a peer-to-peer AI marketplace to improve market access and solve the long tail problem holds significant meaning. Seeing how Bittensor’s concept can change the AI market is something to look forward to.
4. What Challenges Are AI and Blockchain Facing?
4-1. Scalability, scalability, scalability
As mentioned in section 1-2, the development of AI is deeply connected to advancements in data processing capabilities. In the past, AI could only recognize data, not generate (converse) with it. Recognition involves processing unrelated pixels of data in parallel, a task achievable by simply feeding in large amounts of data for learning. However, generative AI, which deals with related data such as grammar and syntax, couldn’t be trained by merely processing large datasets in parallel. This difference meant that while AI technologies like facial and photo recognition advanced rapidly, generative AI took time. However, this dynamic changed in 2017 when Google developed the Transformer neural network architecture, overcoming these issues and leading to a surge in the generative AI market with the release of various products like ChatGPT and SORA.
The characteristics of AI described above contrast sharply with those of blockchain. Blockchain technology aims to solve issues such as censorship as well as data sovereignty and opacity by storing, processing, and recording data based on a consensus algorithm, independent of a centralized authority. Consequently, blockchain inherently faces limitations in data processing capabilities compared to centralized systems. The complex process of countless nodes reaching consensus on data processing is inherently slower than a centralized entity managing the data in one go. Thus, the integration of blockchain and AI is likely to encounter significant scalability challenges. In order for blockchain and AI to merge successfully and achieve mass adoption, scalability issues within blockchain must first be addressed.
- Data Processing and Storage Capacity: AI systems process and learn from massive amounts of data. Integrating AI with blockchain poses a scalability challenge, as storing and processing all AI-related data on the blockchain would be demanding. Blockchain, by design, stores all transaction data across every node in the network, leading to potential scalability issues when handling and storing the vast quantities of data generated by AI.
- Transaction Processing Speed: AI applications require fast data processing speeds. Whether blockchain networks can handle the speed required by AI remains uncertain. Although blockchains like Solana are theoretically capable of handling up to 12,000 transactions per second (TPS), and Aptos could potentially manage more than 160,000 TPS, these figures are still theoretical and fall short of what AI specifications demand. For instance, ChatGPT 2 performed operations at a rate of a quadrillion operations per second for days on end for its training. ChatGPT 3.5 and 4 require even more operations, and the computational needs of future models are yet unknown. Additionally, according to research by the American institute SemiAnalysis, ChatGPT responds to about 160 million data requests per day. This means that in order for an AI model to function on blockchain, they must first address scalability issues.
- Increased Network Complexity: Integrating AI functions into blockchain can increase network complexity, making network maintenance and scalability even more challenging. The computational power needed to execute complex AI algorithms and smart contracts places additional burden on the scalability of blockchain networks.
Final Thoughts
Significant improvements in data processing capabilities brought on the advancement of AI, and efforts continue to enhance the speed and performance of AI models. It’s clear that data processing capability is an indispensable aspect for AI. However, merging blockchain and AI may seem an inefficient choice from a scalability perspective, and needs to be addressed before blockchain can fully serve as a solution for AI. Still, we view fondly of the AI-blockchain merge and foresee blockchain offering solutions to the challenges that accompany the advancement of AI. Blockchain technology has been consistently improving scalability through Dencun upgrades, rollups, and subnets, and the discussion around integrating AI continues. Moreover, recent discussions and developments on ZKML, a combination of Zero-Knowledge Proofs (ZKP) with Machine Learning (ML), are promising. Scalability issues are expected to improve over time, leading to an increase in protocols that integrate blockchain and AI. Specifically, projects like Worldcoin, NEAR Protocol, and Bittensor have already presented their solutions to address the challenges posed by AI. These concepts proposed by these protocols are significant as they indicate their preparations for the challenges of the new era, of which anticipations are reflected in the current hype in the AI sector. As the AI market continues to grow rapidly, this enthusiasm is likely to persist for some time. We hope to soon see the 2024 “AI Summer” after the “DeFi Summer of 2020.”