Introduction
Recently, ChatGPT has gained a great deal of popularity, impressing its users with its capacity to enhance traditional text, work efficiency, and provide concise overviews. Following closely behind is CodeGPT, a GPT-based plugin that further enhances coding efficiency. With the recent release of GPT-4, can it be applied to auditing blockchain and Solidity smart contracts? Based on this question, we conducted various feasibility tests.’
Testing Environment and Methodology
The comparison models used in this test are: GPT-3.5(Web),GPT-3.5-turbo-0301,GPT-4(Web).
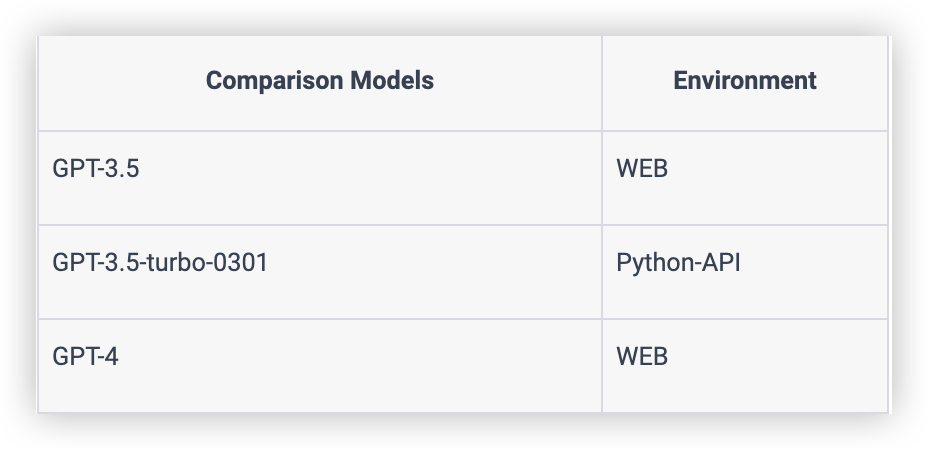
Prompt used in the test: Help me discover vulnerabilities in this Solidity smart contract.
Comparison of Vulnerability Code Snippet Detectio
We performed three rounds of testing. In tests 1 and 2, we utilized historical vulnerability codes commonly encountered in the past as test cases to evaluate the model’s ability to detect fundamental vulnerabilities. In Test 3, we introduced moderately challenging vulnerability codes as the primary test cases.
Test 1:
Example: “Intro to Smart Contract Audit Series: Phishing With tx.orgin”
Vulnerability Code:
Sent to GPT:
GPT-3.5(Web) Response:
GPT-3.5-turbo-0301 Response:
GPT-4(Web) Response:
As you can see from the results, all three models identified critical issues related to tx.origin.
Test 2:
Example: “Intro to Smart Contract Security Audits | Overflow”
Sent to GPT:
GPT-3.5(Web) Response:
GPT-3.5-turbo-0301 Response:
GPT-4(Web) Response:
It is worth noting that both GPT-3.5 (Web) and gpt-3.5-turbo-0301 were able to identify a critical overflow vulnerability, whereas surprisingly, GPT-4 (Web) did not provide any relevant prompt.
Test 3:
Example: “Empty-handed with a White Wolf — Analysis of the Popsicle Hack”
Sent to GPT:
GPT-3.5(Web) Response:
GPT-3.5-turbo-0301 Response:
Looking at the results,, we can see that none of the three versions detected any of the critical vulnerability points.
Summary of Code Snippet Detection
While the GPT models displayed adequate detection capabilities for simple vulnerability code snippets, it falls short when it comes to identifying more complex ones. Throughout the tests, GPT-4 (Web) showcased exceptional readability and a clear output format. However, its ability to audit code does not appear to surpass that of GPT-3.5 (Web) or GPT-3.5-turbo-0301. In some cases, due to the inherent uncertainties in the transformer output, GPT-4 (Web) managed to overlook certain critical issues.
Comparative Detection of Known Vulnerabilities in Full Contracts
To better accommodate the practical requirements of projects during contract audits, we raised the difficulty level by importing contracts with an extensive codebase. This allowed us to comprehensively test the GPT-4 model’s auditing capabilities, as opposed to GPT-3 which has a smaller contextual character limit and thus was not evaluated in this context.
For this instance, we used previous case studies as a test template to simulate real-world scenarios:
Example: “Detailed analysis of the $31 Million MonoX Protocol Hack”.
To initiate the audit, we inputted the complete contract in batches and submitted a vulnerability detection request towards the end of the dialogue.
The following prompt was utilized for this test:
“Here is a Solidity smart contract”
Insert Contract Code
“The above is the complete code,help me discover vulnerabilities in this smart contract.”
As demonstrated, despite GPT-4 having the highest single input character limit, according to the information published by OpenAI, it still encountered contextual challenges due to text overflow during the final vulnerability detection request. Consequently, the model can only identify a portion of the content, rendering it incapable of conducting a thorough contextual audit for large-scale contracts.
Batched Auditing: Unpacked Contracts through Incremental Input and Detection:
Prompt 1:
“Help me discover vulnerabilities in this Solidity smart contract.”
Batch 1 of the contract code.
Prompt 2:
“Help me discover vulnerabilities in this Solidity smart contract.”
Batch 2 of the contract code.
Prompt 3:
“Help me discover vulnerabilities in this Solidity smart contract.”
Batch 3 of the contract code.
It is worth mentioning that GPT-4 failed to identify any critical vulnerability points.
Summary: While the current state of GPT’s capabilities may not be entirely suitable for contract analysis, the potential of AI in this domain remains impressive.
Advantages:
While GPT’s detection capabilities for complex vulnerabilities in contract code may be limited, it has shown impressive partial detection capabilities for basic and simple vulnerabilities. Additionally, once a vulnerability is identified, the model provides an explanation in an easily understandable and user-readable format. This unique feature is especially beneficial for novice contract auditors who require quick guidance and straightforward answers during their initial training phase.
Challenges:
There is a certain amount of variation in GPT’s output for each dialogue, which can be adjusted through API interface parameters. However, the output is still not constant. Although such variability is beneficial for language dialogues and greatly enhances the authenticity of the conversation, it is not ideal for code analysis work. In order to cover multiple possible vulnerability answers that AI may provide, we had to make multiple requests for the same question and compare and filter the results. This inadvertently increases the workload, ultimately undermining the fundamental objective of AI in assisting humans to improve their efficiency.
For instance, we conducted an additional test by running Test 2 of the Comparison of Vulnerability Code Snippet Detection with a slight modification of the function name before generating again.
As we can see, its output results have added some additional content compared to the previous test.
There is still significant room for improvement in its vulnerability analysis capabilities.
It is worth noting that the current (as of March 16, 2024) training models of GPT are unable to accurately analyze and identify critical vulnerability points for slightly complex vulnerabilities.
Despite the current limitations of GPT’s analysis and mining capabilities for contract vulnerabilities, its ability to analyze and generate reports on simple code blocks for common vulnerabilities still sparks excitement among users. With continued training and development of GPT and other AI models, we firmly believe that assisted auditing of large and complex contracts will achieve faster, more intelligent, and more comprehensive outcomes in the foreseeable future. As technological development exponentially improves human efficiency, a transformative shift is imminent. We eagerly anticipate the benefits of AI in enhancing blockchain security and remain vigilant in monitoring the impact of emerging AI products on this vital field. In the visible future, we will inevitably integrate with AI to some extent. May AI and blockchain be with you.